0
AI Is an Existential Threat to Itself
Original article seen at: www.theatlantic.com on June 21, 2023
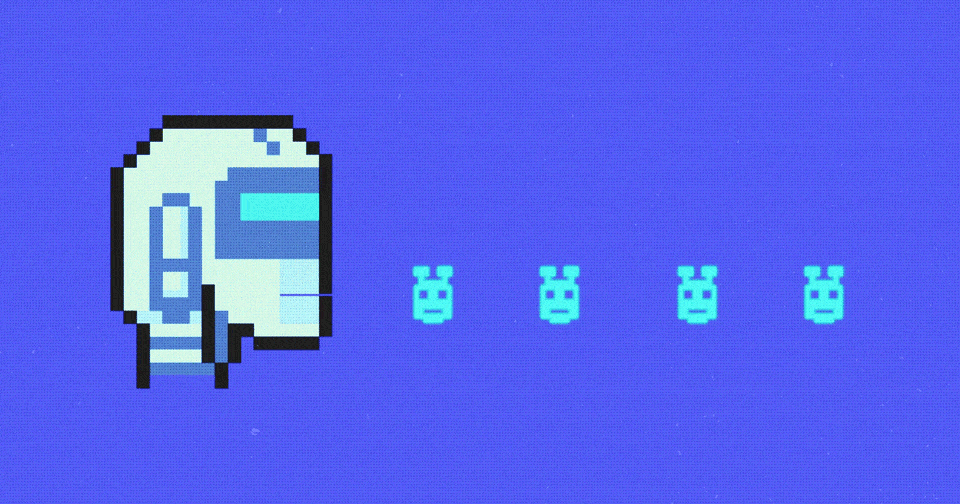
tldr
- π Recursive training of AI on AI-generated data can lead to 'model collapse'.
- π The proliferation of AI-generated content on the web could exacerbate this problem.
- π§ Careful curation of training datasets is crucial to mitigate these risks.
- π AI-generated data could be used to counterbalance existing biases in datasets.
summary
The article discusses the potential risks associated with the recursive training of AI models on AI-generated data. It highlights the phenomenon of 'model collapse', where AI models forget over time, leading to the amplification of errors and biases. The article also points out that the more AI-generated content proliferates on the web, the harder it becomes to distinguish it from human-made content. This could lead to AI models being trained on a mix of human and AI-generated data, potentially exacerbating the problem of model collapse. The article suggests that the solution to this problem lies in carefully curating training datasets and possibly using AI-generated data to counterbalance existing biases.starlaneai's full analysis
The issue of 'model collapse' due to recursive training on AI-generated data is a significant concern for the AI industry. It highlights the need for careful curation of training datasets and the potential use of AI-generated data to counterbalance existing biases. However, implementing these solutions may not be straightforward and could require collaboration among various stakeholders in the AI industry. Furthermore, the proliferation of AI-generated content on the web could exacerbate this problem, making it harder to distinguish AI-generated data from human-made data. This could have implications for the quality and reliability of AI systems, potentially affecting investment decisions in the AI industry. Therefore, addressing this issue is crucial for the continued development and advancement of AI technology.
* All content on this page may be partially written by a clever AI so always double check facts, ratings and conclusions. Any opinions expressed in this analysis do not reflect the opinions of the starlane.ai team unless specifically stated as such.
starlaneai's Ratings & Analysis
Technical Advancement
60 The article discusses a significant issue in AI training, which is a crucial aspect of AI development. However, it does not introduce any new technology or method.
Adoption Potential
30 The discussed issue is relevant to all AI developers, but the proposed solution requires careful implementation and may not be easily adopted by all.
Public Impact
40 The issue has indirect implications for the public, as it affects the quality and reliability of AI systems that people interact with.
Innovation/Novelty
70 The discussion of 'model collapse' due to recursive training on AI-generated data is a relatively novel topic in the AI industry.
Article Accessibility
50 The article uses technical language that may not be easily understood by a general audience.
Global Impact
35 The issue and its potential solutions are globally relevant, as they apply to AI development worldwide.
Ethical Consideration
80 The article extensively discusses the ethical implications of bias amplification in AI systems.
Collaboration Potential
50 The issue requires collaboration among AI developers, researchers, and ethicists to effectively address.
Ripple Effect
60 The discussed issue could have ripple effects on various sectors that rely on AI, as it affects the quality and reliability of AI systems.
Investment Landscape
40 The issue could affect AI investment decisions, as it pertains to the quality and reliability of AI systems.